Premolab.ru
Сайт в процессе разработки
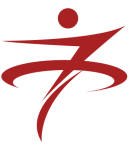
Сделать сайт просто как «раз-два-три»!
Раз. Выбрать и зарегистрировать свободное доменное имя.
Два. Заказать хостинг, выбрав подходящий тарифный план или заказать установку выделенного сервера.
Три. Заказать создание сайта у нашего специалиста. Мы можем предложить вам создание сайта любой сложности.
Первый шаг вы уже сделали, зарегистрировав доменное имя. Следующими шагами будут заказ хостинга и создание сайта.
Второй шаг - заказ хостинга из предлагаемых тарифных планов. Также вы можете заказать у нас установку выделенного сервера.
Шаг третий - создание сайта. Вы можете заказать сайт любой сложности, связавшись с нашим специалистом.
WHOIS – проверка домена
Expired.ru
Список освобождающихся доменов в зоне .RU и .РФ, сервис перехвата доменов. Заявка на регистрацию домена подается через максимально возможный пул регистраторов, что значительно увеличивает ваши шансы.